Aiqun Li1, *, Liaye Li1, Lihua Nong2
1Department of Education, Beibu Gulf University, Qinzhou 535011, China
2Department of International, Nanning Technical College, Nanning 530008, China
*Corresponding email: liaiqun@bbgu.edu.cn
https://doi.org/10.71052/geb2025/WKSX2601
Abstract
Kindergarten teachers are very important guides on the path of young children’s growth. Education is a difficult task, and teaching young children to enjoy learning, to live, and to love art is not enough based on what teachers learn in school alone, and much of it is inappropriate. Early childhood teachers cannot just take books and teach; they must have an attitude of research. It is difficult for teachers to receive good results without an attitude of research, because the work of early childhood education is difficult and complex. Early childhood teachers must coordinate multiple areas to educate young children in a holistic manner, which requires teachers to have strong learning and research skills. At present, there are not many studies on strategies to improve the educational research ability of early childhood teachers, and those that do exist focus more on scientific research, which is somewhat deviated from the educational research needed in kindergartens and can provide limited reference value to teachers, and there is still confusion about how to improve the educational research ability of early childhood teachers. Therefore, this paper proposes a study on the improvement of early childhood teachers’ educational research ability with the technical support of big data. Based on big data and artificial intelligence technology, this paper investigates and analyzes the current situation of kindergarten teachers’ educational research ability, and on this basis, conducts action research to explore the effective path to improve teachers’ educational research ability.
Keywords
Big data technology, Early childhood teachers, Educational research skills, Deep learning
Introduction
The combination of kindergarten education teaching and research is the main expression of the extension and expansion of kindergarten teaching and research in the new situation. At present, the implementation in some early childhood institutions in China is not optimistic and requires reflection to find practical and effective strategies to solve the existing problems [1-3].
The educational research capacity of early childhood teachers can be divided into three levels according to the perspective of research horizon, which are macro and micro levels. Macroscopic educational research is concerned with the construction of basic educational theories and educational fundamentals and is far-sighted and more primarily concerned with conducting educational science research. The practical handbook of educational scientific research defines educational scientific research in this way: a creative cognitive activity aimed at exploring the laws of education by taking the phenomena occurring in the field of education as the object. It is the use of educational theory to study educational phenomena and explore new and unknown laws to solve new problems and situations. Educational research is a continuous, systematic, purposeful, and planned exploration activity. The steps of educational research are identifying a research topic; developing a research plan; implementing research work; and writing a research report. This level of educational research competence refers to the ability to conduct standardized, professional, and systematic educational research. Educational research at the level is thinking about and responding to some meso-level issues or topics in educational management and is designed to solve educational management and decision-making problems. The core of educational research competencies for early childhood teachers is shown in Figure 1.
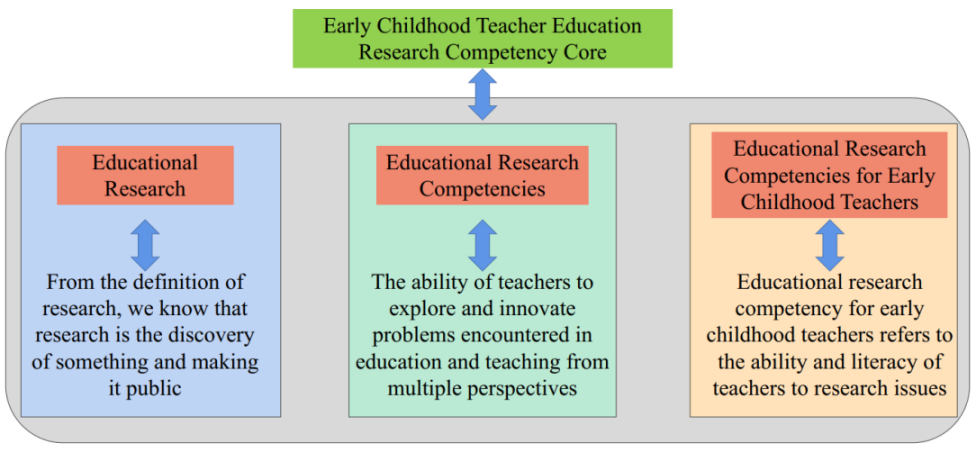
Figure 1. Early childhood teacher education research competency core.
Educational research ability of early childhood teachers refers to the ability and literacy of teachers to research problems, the awareness and ability to link theoretical knowledge with practice, the ability to think openly and independently, and the quality to develop a wide range of interests and conduct permeable learning. It is the ability to be professionally sensitive, reflective, research-minded and cooperative in collective educational activities, and to be proactive in improving one’s work in early childhood education. Big data technology is a new technology that has emerged along with the continuous development of information technology, combined with advanced artificial intelligence and the Internet [4-7]. This technology can well assist people to start the analysis of problems, especially in processing data and screening information, big data has very strong advantages, so applying big data technology to early childhood teachers’ educational research ability and management activities is essential to continuously improve the kindergarten operation Teachers can combine the application of big data technology to collect the daily attendance, learning and living conditions of children, and analyze the shortcomings of teachers’ educational research ability with the help of big data, so as to help teachers take more effective measures to cope with them. In addition, with the development of information technology, parents also want to know the performance of children in kindergartens and various problems at anytime and anywhere, which require kindergartens to actively apply big data technology in the process of running kindergartens, so to continuously improve the information level of kindergarten teachers’ educational research ability is a problem that kindergartens must pay attention to under the current new situation [8].
By constructing an evaluation index system of early childhood teachers’ educational research ability, using the fuzzy comprehensive evaluation method to evaluate early childhood teachers’ research ability in the context of industry-university-research cooperation, and conducting a case study with a kindergarten, we enriched the research system of early childhood teachers’ research ability by proposing corresponding improvement strategies in four aspects, such as strengthening the ability of environment identification [9-10]. The Delphi method was used to construct the index evaluation system, and the hierarchical analysis method was used to determine the index weights, according to which an evaluation model based on five elemental links was proposed, and the evaluation model was applied to a vocational college for a case study, which provided a new research idea for the evaluation of early childhood teachers’ research ability.
By using rough set theory, we constructed an index system of early childhood teachers’ scientific research ability, simplified early childhood teachers’ scientific research indexes by using attribute simplification algorithm, used this to find key factors affecting early childhood teachers’ scientific research ability, and then used association rule algorithm to explore potential rules of early childhood teachers’ scientific research ability, which provided decision support for analyzing early childhood teachers’ scientific research ability and helped to comprehensively investigate early childhood teachers’ scientific research ability. By establishing the scientific research evaluation index system of early childhood teachers in colleges and universities, the clustering algorithm in data mining theory is used to determine the scientific research index, and algorithms such as classification rule-based analysis method and Apriori algorithm are used to mine the association rules affecting the scientific research ability of early childhood teachers. It is used to reflect the research ability of early childhood teachers comprehensively.
Related work
Educational research skills of early childhood teachers
Currently, the pedagogical links between teaching and research are not strong enough to integrate the practical aspects of educational research links. Therefore, a strong linkage mechanism is a prerequisite for effective activity and protection. However, the links between many fields of study and research lack a complete organizational and management mechanism. So-called links are simply the interconnection of several kindergartens through official orders or documents, with inadequate guidance on strategies for scheduling events, advancing them, and assessing their impact, and a lack of a rational linkage system to manage them. The absence of linked teaching and research subjects, in order to accomplish the development goals of teaching and research, requires efforts to promote the collective social responsibility of each early childhood teacher to grow together, but due to our lack of knowledge of the teachers in the actual educational and pedagogical relationships in kindergartens and schools in each region, we also neglect to have an in-depth understanding of the real situation in the relevant teaching and research and pedagogical practices, and are unable to conduct case studies, and in some special places tend to ignore physical teaching theory practice and the real teaching needs of teachers in actual urban and rural schools. Over-emphasis on the power of the joint and single-minded development of large-scale educational activities ignores the actual goal of optimizing the work of kindergarten teaching and research.
The content of linked teaching and research deficiencies teaching, and research articulation is critical, and it is an important factor in improving the quality of actual teaching and learning. However, today in many closely interrelated subject doctrines and technical research topics, almost everything is proposed by the technical planners of professional organizations themselves, while there are certain problems in the main content of these research topic doctrines with obvious content drawbacks. There is often too much emphasis on collaborative learning and research, while also neglecting in-depth study of other aspects of the actual situation. Sometimes although it involves many aspects of activity games, life experience activities, independent development activities, etc., but also because the research is not deep enough, there is an undesirable phenomenon of emphasizing learning over playing, teaching over playing, etc. Too much emphasis is placed on various methods of teaching education research, ignoring the actual details of early childhood education research, which cannot fully reflect the current situation of teaching early childhood school subjects, and cannot give full play to the educational functions of various activities such as teams and groups. At the same time, too much emphasis is placed on learning research, neglecting research on teacher development, and learning resource development, and its linked content is relatively homogeneous, weakening the enthusiasm and initiative of teachers’ participation in the link. There is a lack of reflection on linked teaching and research. In the process of combining education and teaching and research, teachers recognize the importance of teaching and research through various forms of research, but often lack the ability to reflect deeply and effectively. Although the various activities at work provide a platform for teachers to discuss issues, teachers only want to experience the classroom process, and time for reflection and practice is often short and time for discussion is scarce. Even when there is reflection, it is rather superficial and does not provide in-depth study of teaching and research issues.
Big data technology
Early childhood teachers’ educational research skills are the ability to analyze and study a theoretical or practical problem in a scientific way. Scientific research is essentially an innovative activity, a practical activity that explores the objective laws of nature and society and is a driving force for the development of human society. Due to the early childhood teachers’ educational research ability of early childhood teachers has received external attention, how to evaluate the educational research ability of early childhood teachers objectively and effectively has caused extensive research among scholars.
Some scholars have explored the feasibility and effectiveness of the evaluation index system of early childhood teachers’ educational research ability by using fuzzy comprehensive evaluation method, hierarchical analysis (AHP), multi-objective decision making, data envelopment analysis (DEA), principal component analysis (PCA), factor analysis, association rule evaluation method, and gray theory evaluation, and have conducted research on the construction of the evaluation index system. The above scholars generally used the comprehensive evaluation method to analyze the level of educational research ability of early childhood teachers, but less involved in the construction of models and the implementation of prediction functions. Support vector machines were introduced into the multi-level index evaluation system of the independent innovation capability of national defense research institutes to predict their independent innovation capability. The SVM algorithm can deal with small samples, nonlinearity, generalization and high dimensionality, but it takes too long computing time in high dimensional data and often does not predict the true results when the data changes a lot.
The kernel function and parameter tuning are cumbersome. Some scholars have also used neural network algorithms to evaluate early childhood teacher education research ability, such as self-organizing feature mapping network (SOM) network, Hopfield network, probabilistic neural network (PNN), LVQ network, neural network, fuzzy neural network, and BP neural network [18]. Neural networks have their unique features in dealing with nonlinear problems, but the convergence speed is slow, the network structure is complex, the selection of learning parameters is generally determined empirically, and overfitting defects are prone to occur. To sum up, it is necessary to seek an appropriate method to construct a highly reliable model for evaluating early childhood teachers’ early childhood teacher education research ability. Xgboost has good flexibility and accuracy in dealing with this problem, but the drawback of Xgboost is that it has more parameters and affects the prediction performance of the algorithm, so it needs to optimize its tuning process. Therefore, the tuning process needs to be optimized. This paper establishes the GA_XGBoost model based on Xgboost, which takes advantage of the global search and flexibility of the genetic algorithm, making it significantly more optimized than the Xgboost model in terms of parameters, convergence speed and evolutionary results.
Methods
Model architecture
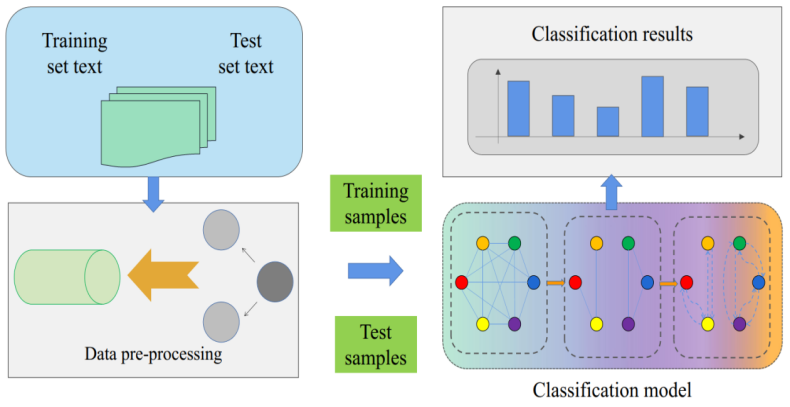
Figure 2. Model structure.
As shown in Figure 2, the research framework for improving the research capacity of early childhood teachers’ education supported by big data technology is an end-to-end deep learning framework, which consists of four parts, namely, word embedding layer, semantic combination layer, convolutional attention layer, and output classification layer. The word embedding layer represents text sequences into a shallow semantic feature matrix with fixed dimensionality by pre-trained word vectors; the semantic combination layer generates a high-level semantic feature matrix by semantically combining the feature vector matrix with a long-short term memory network LSTM; the convolutional attention layer uses a convolutional neural network to extract features from high-level semantic features and generate adaptive attention weights; the output classification layer. The output classification layer is to adjust the feature dimension using the fully connected layer and classify them with SoftMax function.
Local semantic extraction
The role of the local semantic extraction layer is to focus on and extract the important contexts in the global context that are semantically related to the aspect items. LSTM and GRU are good at processing sequential text, and their performance is comparable, but GRU is structurally simpler and has fewer parameters. In this section, new aspect gates and complementary gates are constructed based on GRU, and the AGRU network is proposed so that the model can learn both sequence semantics and select specific aspect information. Intuitively, for each time step, AGRU retains contextual information related to aspect items by aspect feature vectors, while discarding irrelevant contextual information. The AGRU cell computes the context embedding xt, the aspect embedding xa, and the context hiding state ht-1 of the previous moment at time t as shown.
Where rt denotes the reset gate, kt denotes the complementary gate, and zt denotes the update gate, all of which are processed by the sigmoid function; xta denotes the aspect gate, which is controlled by the ReLU function.
ReLU (x) = max(0,x) → → → → → (2)
Due to the unilateral suppression of this function, the feature vector after the interaction between aspect features and context features is sparser after its processing, which is conducive to aspect – related context feature extraction. lta and lt denote the linear transformations of xta and xt, respectively, which are complementary to the dimensional space of AGRU units. Their expressions are shown.
ltα = Wlα(xtα) → → → → → → → (3)
lt = Wl(xt)
denotes the candidate hidden state, which is controlled by the aspect gate, complementary gate, and reset gate along with the tanh function to update and retain the current input, thus selecting the aspect-oriented sequence context. w1a,w1 are the learnable weight matrices and deviation vectors.
Feature learning layer
The syntactic dependency tree can be transformed into a graph in which the nodes represent the words in each sentence, the edges represent the dependency labels between words, and the structure of the graph is represented by the adjacency matrix. To increase the closeness between the context and a particular aspect, the adjacency matrix, e, is calculated as shown in equation.
eij = dij * (tij + 1) → → → → → → → (4)
Where dij is the schematic function and a dependency relationship between node I and node j, dij is 1, otherwise it is 0. When node i and node j are aspect words, tij is 1, otherwise it is 0. RGAT has a multi-layer architecture, under the constraint of the adjacency matrix, each layer combines node features and dependent label features in the neighborhood based on multi-headed attention to the attention calculation and aggregation process to achieve each node vector. The output hidden state of AGRU is used as the initial hidden state of RGAT, and as the number of layers increases, the stacked hidden state of all nodes is generated iteratively. Taking layer l as an example, the update process of node I under the s-the attention head of the layer is shown in equation.
hils = σ (j∈Ni αijls (· (Wvls(l-1)s hj(l-1)s + Wrlsij rij)) → → → → → (5)
Where · hils is the updated vector representation of node i, hj(l-1)s is the vector representation of neighbor node j in the upper layer, rij is the dependency label representation between node i and j, and · Wvls, Wrls is the learnable weight matrix. αijls is the normalized weight coefficient, which represents the attention fraction of node i to neighbor node j.
Where, d is the scaling factor of one attention head and Wqls, Wkls, Wklsr is the learnable weight matrix. The multi – head attention is learned according to equation, and different vector update representations about node i are obtained after several transformations, and the multiple vector results are stitched together to obtain the final target node representation hi1s, layer – 1.
Deep interaction fusion
The syntactic context obtained from the upper layer network belongs to coarse-grained text representation. On the one hand, RGAT models the dependencies between other words in addition to learning the correspondence between specific aspects and early childhood teacher education research competency words. On the other hand, the dependency tree parsing of complex sentences is not accurate enough and will mislead the model to focus on useless information and introduce additional noise. Compared with the syntactic context, the weight of redundant information in the local semantics is smaller, but it still affects the model performance.
The pooled vectors are projected into the space of target K classes using a fully connected layer to calculate the probability of each early childhood teacher education research competency assessment.
Experiments and results
Experiment setup
Confrontation sample validity validation was conducted on different network models, and the information related to the dataset was summarized by statistically analyzing multiple types of textual datasets for early childhood teacher education research competency assessment. The dataset for the experiment was constructed in this paper using multiple types of data, with an average of 6,000 early childhood teacher education research competency reports of each type. Since this experiment only needed to verify the comment statements that contained polyphonic words belonging to early childhood teachers’ educational research competency tendencies, after manual screening, 5886 statements containing polyphonic words were left in the comments with the same proportion of positive and negative samples.
Adversarial sample data were generated using PGAS, and the adversarial sample data, passed into traditional models such as long and short-term memory networks and convolutional neural networks and some of the latest early childhood teacher education research competency assessment models to test the effectiveness of the generated samples. To verify the effectiveness of the proposed PGAS, the adversarial samples were first generated, and these adversarial samples were used as input to implement a black-box attack on the existing state-of-the-art early childhood teacher education research competency assessment models. The measurement of the effectiveness of the PGAS attack is reflected by the degree of decrease in the accuracy of the neural network model for the detection of the adversarial samples; the more the decrease in accuracy, the more effective the attack is. The configuration of the experimental environment is shown in Table 1.
Table 1 Software and hardware environment configuration
Parameters | Configuration |
Operating system | Ubuntu 20.04.2 LTS |
CPU | Inter Core i9-10900KF |
CPU memory | 62.7 G |
GPU | GeForce RTX 3080 |
GPU memory | 24G |
Programming language | Python |
Programming platforms | PyCharm |
Graphics acceleration environment | CUDA11.2, CuDNN7.6 |
Deep learning framework | Potarch 1.7.0, Torch vision 0.8.1 |
Experimental results
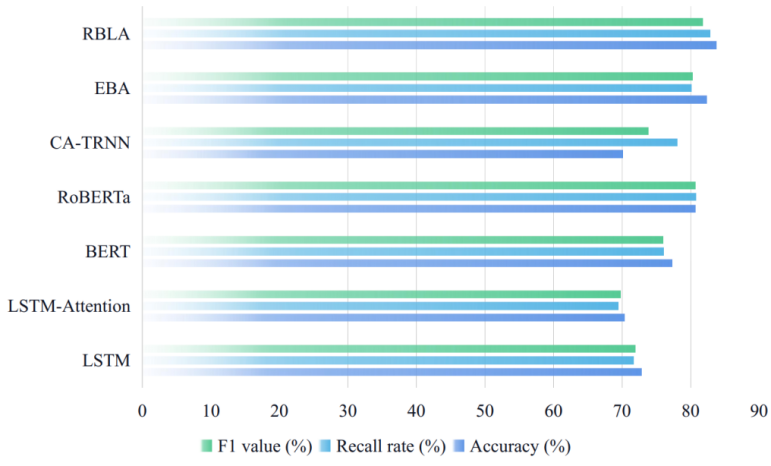
Figure 3. Comparison of experimental results.
To verify the overall performance of the RBLA model in the classification of implicit early childhood teacher education research competency, comparison experiments were conducted with the six models mentioned above to test their accuracy, recall and F1 values, and their experimental results are shown in Figure 3. From Figure 3 4, the RBLA model has 3.07%, 2.12% and 1.01% higher precision, recall and F1 values, respectively, compared with the RoBERTa model, which has the best performance among the four baseline models of LSTM-Attention, LSTM, BERT and RoBERTa. This indicates that the RBLA fusion model proposed in this paper has better performance on the implicit early childhood teacher education research competency analysis task compared to the typical baseline model. In addition, compared with the EBA model, which is the best classification model among the two current mainstream implicit early childhood teacher education research competency analysis models, CA-TRNN and EBA, the RBLA model has 1.44%, 2.72%, and 1.42% higher precision, recall, and F1 values than the EBA model, respectively.
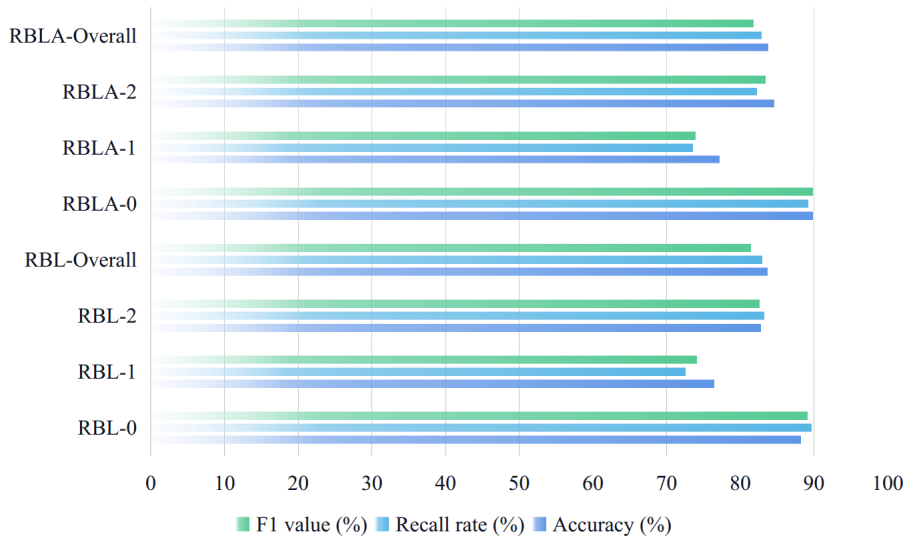
Figure 4. Comparison of the results of the assessment of early childhood teachers’ educational research ability.
In this paper, the early childhood teacher education research competency tendency categories of implicit early childhood teacher education research competency tests are defined as neutral, positive, and derogatory, which are represented by the labels 0, 1, and 2, respectively. To investigate the influence of the attention mechanism in the RBLA model approach on the classification of early childhood teacher education research competency, we compared the performance of the RBLA model and the RBL (Roberta Bi-LSTM) model without the attention mechanism for the classification of implicit early childhood education research competency, and the experimental results are shown in Figure 4.
As can be seen from Figure 4, the RBLA model proposed in this paper was 1.63%, 0.71%, and 1.73% more accurate than the RBL model in classifying three types of early childhood teachers’ educational research competencies: neutral, positive, and derogatory, respectively. Similarly, compared to the RBL model, the RBLA model was 0.72% and 0.78% higher in F1 values for the neutral ECE teacher education research competency classification and the pejorative ECE teacher education research competency classification, respectively, and 1.04% higher in recall for the positive ECE teacher education research competency classification. Although the RBLA model was slightly lower than the RBL model in the recall rate of the neutral early childhood teacher education research competency and derogatory early childhood teacher education research competency classifications and the F1 value of the positive early childhood teacher education research competency classification, in terms of overall performance, the RBLA model was higher than the RBL model in terms of accuracy rate and F1 value.
Conclusion
Today is an era of pursuing excellence, and the desire for excellence in all walks of life has become stronger and stronger, and the source of these talents undoubtedly comes from education, and excellence in education cannot be separated from excellence in teachers. With the deepening of basic education reform and the call of the times for excellent early childhood teachers, early childhood teachers’ textbook research literacy has become a key literacy for teachers, which is important to promote their professional growth and enhance the innovation level of education and teaching. The pre-service training of early childhood teachers’ educational research literacy has the significance of laying the foundation for the growth of excellent early childhood teachers in the future and promoting research-based learning among teacher trainees. How to cultivate early childhood teachers’ educational research literacy at the pre-service stage is an issue worthy of research and exploration.
In the early childhood education stage, the application of big data technology can well change the current teaching status quo, improve the scientific nature of kindergarten teaching and management activities, and enable kindergartens to obtain more targeted information. At the same time, the application of big data in kindergarten early childhood teachers’ educational research ability can also enable early childhood teachers to implement targeted teaching by combining the characteristics of each child, so that each early childhood teacher’s educational research ability can be fully developed. Therefore, kindergartens should continuously increase the learning and application of big data technology in the process of running kindergartens, make full use of advanced big data technology to improve preschool education informatization, and create favorable conditions for every early childhood teacher to actively participate in the improvement of early childhood teachers’ educational research ability.
Acknowledgements
This work was supported by Guangxi Education Science “14th Five-Year Plan” 2021 Special Project for Capital Construction Research (Project No. 2021ZJY1968); Guangxi Education Science Planning 2021 Special Project for Preschool Vocational Education Schools (Project No. 2021ZJY808).
Reference
[1] Danniels, E., Pyle, A. (2024) Promoting inclusion in play for students with developmental disabilities: Kindergarten teachers’ perspectives. International Journal of Inclusive Education, 28(5), 457-474.
[2] Aal Ismail, H., More, C., Baker, J., Huff, S. (2022) Integrating augmentative and alternative communication into a stay-play-talk program in preschool. Teaching Exceptional Children, 55(2), 122–130.
[3] Anderson-Butcher, D., Bates, S., Lawson, H. A., Childs, T. M., Iachini, A. L. (2022) The community collaboration model for school improvement: A scoping review. Education Sciences, 12(12), 91
[4] Bower, C. A., Zimmermann, L., Verdine, B. N., Pritulsky, C., Golinkoff, R. M., Hirsh-Pasek, K. (2022) Enhancing spatial skills of preschoolers from under-resourced backgrounds: A comparison of digital app vs. concrete materials. Developmental Science, 25(1), e13148.
[5] Helsabeck, N. P., Justice, L. M., Logan, J. A. R. (2022) Assessing Fidelity of Implementation to a technology-mediated early intervention using process data. Journal of Computer Assisted Learning, 38(2), 409–421.
[6] Mishra, M., Warr, M., Islam, R. (2023) TPACK in the age of ChatGPT and Generative AI. Journal of Digital Learning in Teacher Education, 39(4), 235–251
[7] Zhang, L., Li, H. (2023) Big data analytics for early childhood education: Enhancing teacher training and classroom practices. Journal of Educational Data Mining, 15(1), 56–78.
[8] Li, Y. (2023) The role of early childhood teachers in shaping educational quality: A review of current practices and future directions. Early Childhood Education Journal, 51(3), 456–468.
[9] Gong, Q. (2025) Research on the mind and characteristics of the constructors of the autonomous knowledge system in Chinese higher education. China Higher Education Research, 41(2), 59-67.
[10] Liu, Z., Zhang, Y. (2025) Artificial intelligence in education: Empowering teachers and students in the digital age. Modern Educational Technology, 2025(2).
Share and Cite
Li, A., Li, L., Nong, L. (2024) Research on the Improvement of Early Childhood Teachers’ Educational Research Ability Supported by Big Data Technology. Global Education Bulletin, 1(1), 1–10. https://doi.org/10.71052/geb2025/wksx2601